This article is available in:
日本語
Introduction
In my previous article, I discussed the theory of logistic regression.
This time, we will implement it using Python.
Also, the following code works with Google Colab.
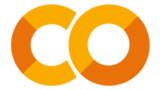
Data Set Preparation
The data used as an example is the iris dataset. The iris dataset consists of petal and sepal lengths for three varieties: Versicolour, Virginica, and Setosa.
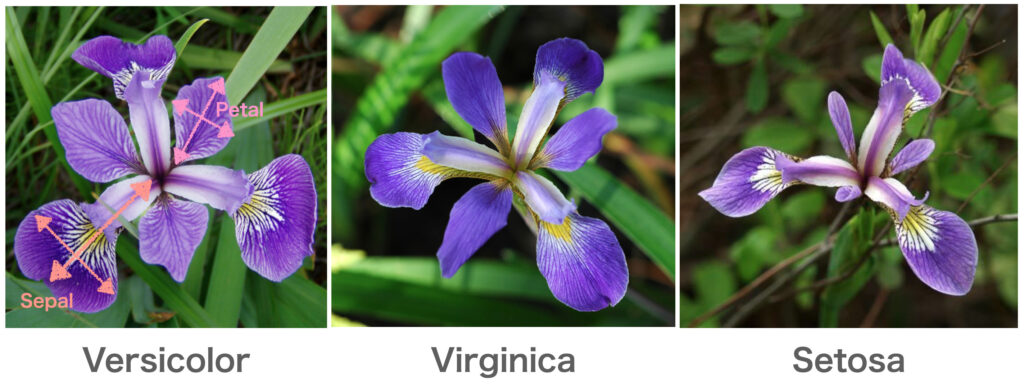
Let’s read the iris dataset using the scikit-learn library.
import numpy as np
import pandas as pd
from sklearn.datasets import load_iris
iris = load_iris() # Loading iris datasets
df_iris = pd.DataFrame(iris.data, columns=iris.feature_names)
df_iris['class'] = iris.target
df_iris
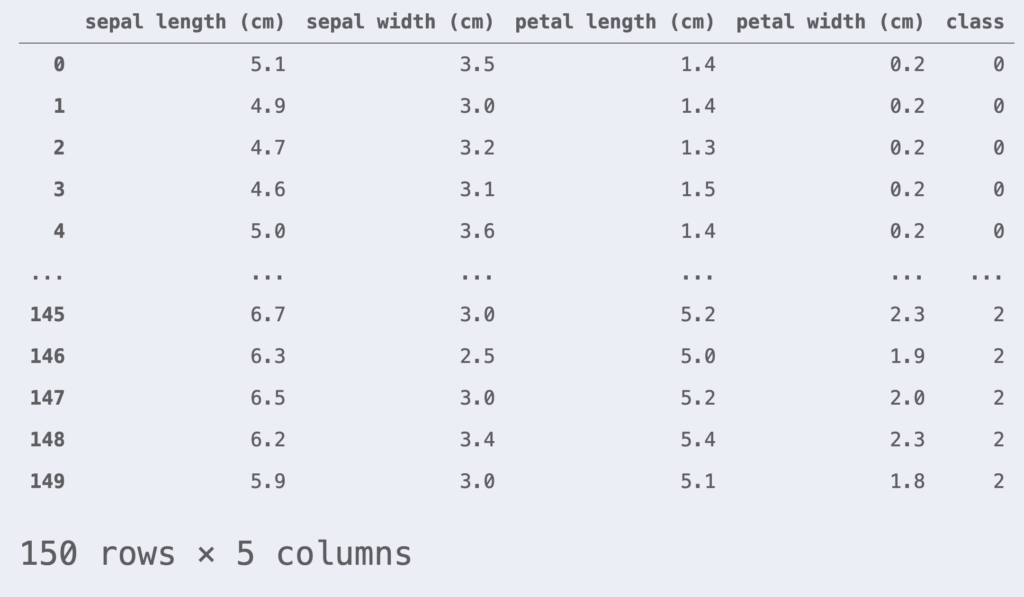
This time we will perform a binary logistic regression classification, focusing only on data with class = 0, 1. For simplicity, we assume that the two features are petal length and petal width.
df_iris = df_iris[df_iris['class'] != 2] # Get only data with class = 0, 1
df_iris = df_iris[['petal length (cm)', 'petal width (cm)', 'class']]
X = df_iris.iloc[:, :-1].values
y = df_iris.iloc[:, -1].values
The data set is standardized to have a mean of 0 and a standard deviation of 1.
from sklearn.preprocessing import StandardScaler
# Generate instances of standardization (converted to mean=0, standard deviation=1)
sc = StandardScaler()
X_std = sc.fit_transform(X)
To evaluate the generalization performance of the model, the data set is split into a training data set and a test data set. In this case, we split the training data at a ratio of 80% and the test data at a ratio of 20%.
from sklearn.model_selection import train_test_split
X_train, X_test, y_train, y_test = train_test_split(X_std, y, test_size=0.2, random_state=1, stratify=y)
The plot class should also be defined here.
# Define plot classes for classification boundaries
import matplotlib.pyplot as plt
from matplotlib.colors import ListedColormap
class DecisionPlotter:
def __init__(self, X, y, classifier, test_idx=None):
self.X = X
self.y = y
self.classifier = classifier
self.test_idx = test_idx
self.colors = ['#de3838', '#007bc3', '#ffd12a']
self.markers = ['o', 'x', ',']
self.labels = ['setosa', 'versicolor', 'virginica']
def plot(self):
cmap = ListedColormap(self.colors[:len(np.unique(self.y))])
# Grit point generation
xx1, xx2 = np.meshgrid(
np.arange(self.X[:,0].min()-1, self.X[:,0].max()+1, 0.01),
np.arange(self.X[:,1].min()-1, self.X[:,1].max()+1, 0.01))
# Find the predicted value for each meshgrid
Z = self.classifier.predict(np.array([xx1.ravel(), xx2.ravel()]).T)
Z = Z.reshape(xx1.shape)
# Plotting Contour Lines
plt.contourf(xx1, xx2, Z, alpha=0.2, cmap=cmap)
plt.xlim(xx1.min(), xx1.max())
plt.ylim(xx2.min(), xx2.max())
# Plot data points by class
for idx, cl, in enumerate(np.unique(self.y)):
plt.scatter(
x=self.X[self.y==cl, 0], y=self.X[self.y==cl, 1],
alpha=0.8,
c=self.colors[idx],
marker=self.markers[idx],
label=self.labels[idx])
# Emphasis on test data
if self.test_idx is not None:
X_test, y_test = self.X[self.test_idx, :], self.y[self.test_idx]
plt.scatter(
X_test[:, 0], X_test[:, 1],
alpha=0.9,
c='None',
edgecolor='gray',
marker='o',
s=100,
label='test set')
plt.legend()
\begin{align*}
\newcommand{\mat}[1]{\begin{pmatrix} #1 \end{pmatrix}}
\newcommand{\f}[2]{\frac{#1}{#2}}
\newcommand{\pd}[2]{\frac{\partial #1}{\partial #2}}
\newcommand{\d}[2]{\frac{{\rm d}#1}{{\rm d}#2}}
\newcommand{\T}{\mathsf{T}}
\newcommand{\(}{\left(}
\newcommand{\)}{\right)}
\newcommand{\{}{\left\{}
\newcommand{\}}{\right\}}
\newcommand{\[}{\left[}
\newcommand{\]}{\right]}
\newcommand{\dis}{\displaystyle}
\newcommand{\eq}[1]{{\rm Eq}(\ref{#1})}
\newcommand{\n}{\notag\\}
\newcommand{\t}{\ \ \ \ }
\newcommand{\argmax}{\mathop{\rm arg\, max}\limits}
\newcommand{\argmin}{\mathop{\rm arg\, min}\limits}
\def\l<#1>{\left\langle #1 \right\rangle}
\def\us#1_#2{\underset{#2}{#1}}
\def\os#1^#2{\overset{#2}{#1}}
\newcommand{\case}[1]{\{ \begin{array}{ll} #1 \end{array} \right.}
\newcommand{\s}[1]{{\scriptstyle #1}}
\definecolor{myblack}{rgb}{0.27,0.27,0.27}
\definecolor{myred}{rgb}{0.78,0.24,0.18}
\definecolor{myblue}{rgb}{0.0,0.443,0.737}
\definecolor{myyellow}{rgb}{1.0,0.82,0.165}
\definecolor{mygreen}{rgb}{0.24,0.47,0.44}
\end{align*}
Full-scratch implementation
Logistic regression is implemented in full scratch. From the previous results, the loss function $J$ of the logistic regression was
\begin{align} J =\, – \sum_{i=1}^n \{ y^{(i)}\log p^{(i)} + (1 – y^{(i)}) \log (1-p^{(i)}) \}. \end{align}
And the update rule for the parameter $\bm{w}$ by the steepest descent method was
\begin{align*}
\bm{w}^{[t+1]} = \bm{w}^{[t]} – \eta\pd{J(\bm{w})}{\bm{w}}.
\end{align*}
The $n$ observed $p$ dimensional data are
\begin{align*}
X = \mat{1 & x_1^{(1)} & x_2^{(1)} & \cdots & x_p^{(1)} \\ 1 & x_1^{(2)} & x_2^{(2)} &\cdots & x_p^{(2)} \\ \vdots & \vdots & \vdots & \ddots & \vdots \\ 1 & x_1^{(n)} & x_2^{(n)} &\cdots & x_p^{(n)}\\}.
\end{align*}
Let $n$ objective variables $y^{(i)}$ and response probabilities $p^{(i)}$ be $n$ and $p^{(i)}$, respectively.
\begin{align*}
\bm{y} = \(y^{(1)} , y^{(2)} , \dots , y^{(n)}\)^\T, \t \bm{p} = \(p^{(1)} , p^{(2)} , \dots , p^{(n)}\)^\T
\end{align*}
The $\pd{J(\bm{w})}{\bm{w}}$ could be written as follows.
\begin{align}
\pd{J(\bm{w})}{\bm{w}} =\large X^\T(\bm{p}\, -\, \bm{y}).
\end{align}
Based on the above, we will implement logistic regression.
class LogisticRegression:
"""Logistic regression run class
Attributes
----------
eta : float
epoch : int
random_state : int
is_trained : bool
num_samples : int
num_features : int
w : NDArray[float]
costs : NDArray[float]
Methods
-------
fit -> None
Fitting parameter vectors for training data
predict -> NDArray[int]
Return predicted value
"""
def __init__(self, eta=0.01, n_iter=50, random_state=42):
self.eta = eta
self.n_iter = n_iter
self.random_state = random_state
self.is_trained = False
def fit(self, X, y):
"""
Fitting parameter vectors for training data
Parameters
----------
X : NDArray[NDArray[float]]
Training data: (num_samples, num_features) matrix
y : NDArray[int]
Teacher labels for training data: ndarray of (num_features, )
"""
self.num_samples = X.shape[0]
self.num_features = X.shape[1]
rgen = np.random.RandomState(self.random_state)
# Initialize parameter vector using normal random numbers
self.w = rgen.normal(loc=0.0, scale=0.01, size=1+self.num_features)
self.costs = [] # Array to store the values of the loss function at each epoch
# Update parameter vectors
for _ in range(self.n_iter):
net_input = self._net_input(X)
output = self._activation(net_input)
# Eq.(2)
self.w[1:] += self.eta * X.T @ (y - output)
self.w[0] += self.eta * (y - output).sum()
# Eq.(1)
cost = (-y @ np.log(output)) - ((1-y) @ np.log(1-output))
self.costs.append(cost)
# Flag the completion of the study.
self.is_trained = True
def predict(self, X):
"""
Return predicted value
Parameters
----------
X : NDArray[NDArray[float]]
Data to predict: (any, num_features) matrix
Returens
-----------
NDArray[int]
0 or 1 (any, ) ndarray
"""
if not self.is_trained:
raise Exception('This model is not trained.')
return np.where(self._activation(self._net_input(X)) >= 0.5, 1, 0)
def _net_input(self, X):
"""
Calculate the inner product of data and parameter vectors
Parameters
--------------
X : NDArray[NDArray[float]]
Data: (any, num_features) matrix
Returns
-------
NDArray[float]
Value of inner product of data and parameter vector
"""
return X @ self.w[1:] + self.w[0]
def _activation(self, z):
"""
Activation function (sigmoid function)
Parameters
----------
z : NDArray[float]
(any, ) ndarray
Returns
-------
NDArray[float]
"""
return 1 / (1 + np.exp(-np.clip(z, -250, 250)))
Now let’s check the implementation using the iris dataset.
# Learning a logistic regression model
lr = LogisticRegression(eta=0.5, n_iter=1000, random_state=42)
lr.fit(X_train, y_train)
# 訓練データとテストデータを結合
X_comb = np.vstack((X_train, X_test))
y_comb = np.hstack((y_train, y_test))
# プロット
dp = DecisionPlotter(X=X_comb, y=y_comb, classifier=lr, test_idx=range(len(y_train), len(y_comb)))
dp.plot()
plt.xlabel('petal length [standardized]')
plt.ylabel('petal width [standardized]')
plt.show()
The decision curve could be plotted in this way.
Implementation using scikit-learn
Using scikit-learn, it is very easy to perform logistic regression.
from sklearn.linear_model import LogisticRegression
# Create an instance of logistic regression
lr = LogisticRegression(C=1, random_state=42, solver='lbfgs')
# Model Learning
lr.fit(X_train, y_train)
Let’s check the implementation using the iris dataset.
# Combine training data with test data
X_comb = np.vstack((X_train, X_test))
y_comb = np.hstack((y_train, y_test))
# plot
dp = DecisionPlotter(X=X_comb, y=y_comb, classifier=lr, test_idx=range(len(y_train), len(y_comb)))
dp.plot()
plt.xlabel('petal length [standardized]')
plt.ylabel('petal width [standardized]')
plt.show()
The decision curve could be plotted like this in scikit-learn.
You can try the above code here▼.
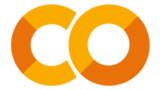